Meetings and Events
Spring 2013
Vol. 8, Issue 1
Winter 2013
Vol. 7, Issue 2
Spring 2012
Vol. 7, Issue 1
Spring 2011
Vol. 6, Issue 1
Fall 2011
Vol. 6, Issue 2
Spring 2010
Vol. 5, Issue 1
Fall 2010
Vol. 5, Issue 2
Spring 2009
Vol. 4, Issue 1
Fall 2009
Vol. 4, Issue 2
Spring 2008
Vol. 3, Issue 1
Fall 2008
Vol. 3, Issue 2
Fall 2007
Vol. 2, Issue 2
Winter 2007
Vol. 2, Issue 1
Summer 2006
Vol. 1, Issue 2
A Conversation With
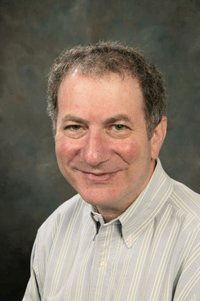
Eric “Rocky” Feuer, Ph.D.
Chief
Statistical Research and Applications Branch
Surveillance Research Program
Division of Cancer Control and Population Sciences
Q: What are the main goals of the Cancer Intervention and Surveillance Modeling Network (CISNET)?
There are three main goals: 1) to understand the impact of cancer control interventions – that includes screening, treatment, and prevention – on current and future trends in U.S. cancer incidence and mortality; 2) to extrapolate evidence from randomized, controlled trials and epidemiologic and observational studies in order to determine the most efficient and cost-effective strategies for implementing technologies in the U.S. population; and 3) to be responsive to the challenges due to the increased pace of new technology by helping to determine which new technologies are the most promising when scaled up to the population level. The cancer sites included so far are breast, prostate, colorectal, and lung.
Q: What is simulation modeling and how is it utilized in cancer control and surveillance research?
Simulation modeling is synthesizing disparate bits of information to simulate real and hypothetical experiences in the U.S. population in order to answer questions that would be difficult to address otherwise. We utilize data from randomized controlled trials, meta-analyses, observational studies, national surveys, cancer registries, and studies of practice patterns to evaluate the past and potential future impact of cancer control interventions in prevention, screening, and treatment.
Q: Translational research is a priority at the National Cancer Institute. Can you give us an example of a successful CISNET translational project outcome?
Breast cancer mortality fell by around 25% in the U.S. between 1989 and 2000. Before that, the death rate had been very flat. One thing people wanted to know was what caused it to decline. The two major factors possibly responsible for the remarkable decline were the introduction of both screening mammography and adjuvant therapy (both multi-agent chemotherapy and hormonal therapy) for breast cancer during the period 1975-2000.
At the time, there were eight randomized controlled studies of mammography screening. In 2000, the Cochrane Collaboration group in the United Kingdom performed a meta-analysis of these studies and threw out all of the studies they felt had flaws in their randomization. A meta-analysis of the two remaining trials showed no effect of screening on both breast cancer and all-cause mortality. Their conclusions caused a huge controversy in the U.S. and elsewhere. We’ve been giving all these women mammograms, and it doesn’t do a thing? The “gold standard” of evidence in medicine is randomized clinical trials, and we’d already done those studies for mammography. We probably weren’t going to do many, if any, more. We asked ourselves where do we go from here?
By coincidence, this controversy erupted just when CISNET was getting started. At that time, we had seven grantees for the new program doing breast cancer modeling. They were developing models to simulate the lives of women with respect to their “natural history” of breast cancer- i.e. women are born, some develop breast cancer, some of those breast cancers grow large enough to become screening detectable, and eventually large enough to cause symptoms. When the cancer is diagnosed, it is treated, and the woman eventually dies of either breast cancer or another cause. Because much of the natural history of breast cancer is never observed, it must be modeled based on indirect evidence, and there are many different approaches to doing this.
One innovation of the approach employed in CISNET is the use of a comparative modeling approach, which explores differences between models in a systematic way. Comparative modeling produces a range of results across models and, when consensus can be reached, greatly enhances the credibility of modeling results by highlighting their reproducibility. The CISNET models shared common input with respect to the dissemination of mammography and adjuvant therapy in the U.S. population.
We can simulate through CISNET what happened in the U.S. population during the period when breast cancer deaths fell. We did and found that – across all seven models –it would’ve been very difficult to explain the entire decline in breast cancer mortality just by changes in treatment alone. On average, about half the decline was due to mammography and half due to adjuvant therapy. This is not definitive evidence, but it is strong evidence, using modeling and population data when we’d exhausted all other means. Our findings were published in the New England Journal of Medicine and were reported on the front page of the New York Times.
Q: Do you see any potential opportunities for applying CISNET’s simulation modeling approaches to gain a better understanding of the phenomenon of complementary and alternative medicine (CAM) use by cancer patients? If so, can you describe a specific example?
OCCAM (Office of Cancer Complementary and Alternative Medicine) Director Dr. Jeffrey White gave a presentation at the CISNET meeting in January 2010. I asked him to provide some examples of CAM interventions with the strongest evidence, which might get researchers and modelers in CISNET thinking about how they could take those CAM approaches and incorporate them into their models. The two examples Dr. White proposed were: 1) impact of diet and exercise on breast and colon cancer survival; and 2) impact of dietary antioxidants on lung cancer survival.
Dr. White gave an overview of the results of the previous clinical trials. The studies didn’t find huge improvements in survival, but the results were statistically significant. The studies seem to have been done in a fairly rigorous way.
Then, the question is what can CISNET do? These CAM interventions are things that can potentially be done fairly inexpensively. If their use was scaled up to the population level, how many person-years of life might be saved? What is the cost per person-year of life (or quality-adjusted person years of life) saved to put some of these CAM strategies on the same scale as other standard medical interventions used in cancer? We could see how cost-effective these CAM interventions might be. One unique aspect of some CAM interventions is that they not only have a potential beneficial impact on cancer mortality but also have a potential positive impact on other causes of mortality. Thus, these interventions infer a double benefit, which modeling can quantify.
Q: Does CISNET have any current or upcoming projects related to CAM?
We don’t currently have any projects related to CAM in cancer. However, we are open to future collaborative opportunities. We’re in the midst of renewing the funding for CISNET, and there may be future opportunities for supplemental funding to facilitate these types of collaborations. CISNET can also work with OCCAM to develop what would be the right prototype opportunity to do some modeling in the CAM field. It would be nice to get one very good case example, like the researchers who studied diet and exercise interventions, who could team up with a CISNET group and model some of this. It might be a nice proof-of-principle of what modeling could add to some of the CAM research being done.
More on CISNET can be found at http://cisnet.cancer.gov/.